Introduction
Introduction
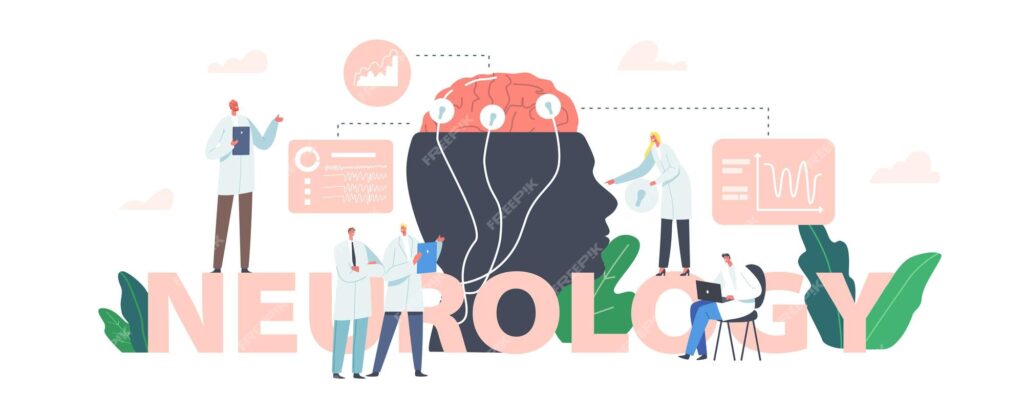
Introduction
Introduction
The Importance of Genetic Factors in NDDs
Twin and family studies have shown that NDDs have a significant genetic component. Heritability estimates for ASD and ID are greater than 0.5. This genetic contribution has led to the use of prenatal genetic testing, such as whole exome or genome sequencing, to identify potentially harmful genetic variations. Whole exome sequencing, in particular, has proven to be a cost-effective method for identifying coding variants in genes that play a crucial role in neurodevelopment.
Identifying NDD Risk Genes
Previous studies have identified many monogenic forms of NDDs, highlighting the polygenic nature of most NDD diagnoses. Rare de novo mutations, which are observed at a significantly higher rate in NDD cases compared to unaffected individuals, have led to the identification of many candidate NDD risk genes. These de novo mutations can be single nucleotide variants, insertions and deletions, or copy number variants. Individuals affected by NDDs have a higher burden of non-synonymous de novo mutations compared to unaffected individuals.
Early Prediction of NDDs
Early prediction of NDDs is crucial for parents to make informed decisions about early intervention treatments. However, most NDD cases cannot be predicted using de novo coding variation alone, as the majority of NDD-associated variants are likely to reside in non-coding regions involved in gene regulation. Currently, only a small fraction of ASD and ID/DD cases have de novo coding variants, and the rate of such variants in the general population is significantly lower.
The Role of De Novo Coding Variation in Early Prediction
Despite the polygenic nature of NDDs, focusing on un-inherited, de novo mutations that disrupt protein coding sequences allows for the early prediction of a small subset of cases with low false positive rates. The early prediction of NDDs requires a very low false positive rate due to potential negative consequences, such as the costs associated with early intervention treatments. The shallow neural net (SNN) model, which incorporates de novo LGD (likely gene-disruptive) mutations, constraint, and conservation data, has shown promising results in achieving a higher true positive rate at very low false positive rates compared to traditional classification models.
Methods for Early Prediction of NDDs
To distinguish NDD cases from unaffected controls using de novo coding variation, LGD and missense variants were retrieved from denovo-db. These variants were incorporated into LGD-specific and missense-specific feature matrices, along with gene score features such as pLI, LOEUF, RVIS, and phastCons. The SNN model, trained on these feature matrices, achieved higher TPR at FPR < 0.01 compared to baseline models such as random forest, support-vector machine, and logistic regression. An ensemble model that combined predictions from different models further improved TPR at low FPR values.
Integration of Missense and LGD-Specific Models
While missense variation alone is a poor predictor of NDD cases, the combination of missense and LGD-specific predictions improved the identification of cases with both types of variants. By taking the maximum predicted probability from separately trained missense- and LGD-specific models, a combined prediction was able to capture a greater fraction of cases at low FPR.
NDD Gene Ranking
The SNN models trained on LGD- and missense-specific variation were also used to rank genes according to their relative importance in NDD risk. Artificial samples were generated with a single de novo variant in a unique gene, and the predicted probability from the SNN models was used to rank the genes. The ranking of genes based on their importance to NDDs can help identify candidate NDD genes that are susceptible to de novo coding variation.
Conclusion
In conclusion, the early prediction of NDDs using de novo coding variation is challenging due to the polygenic nature of these disorders. However, focusing on un-inherited, de novo mutations that disrupt protein coding sequences can allow for the early prediction of a small subset of cases with low false positive rates. The SNN model, along with gene score features, has shown promising results in accurately classifying NDD cases at very low false positive rates. Further research and the integration of additional biomolecular signatures are needed to increase the reach of early prediction to a larger fraction of NDD cases.
Keywords: Neurodevelopmental disorders, NDDs, genetic basis, early prediction, de novo coding variation, shallow neural net (SNN), missense variation, LGD-specific variation, gene ranking